mirror of
https://github.com/finegrain-ai/refiners.git
synced 2024-11-24 15:18:46 +00:00
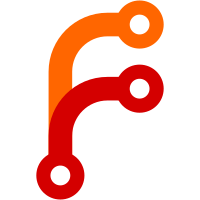
Note: dense prompts (i.e. masks) support is still partial (see MaskEncoder) Co-authored-by: Cédric Deltheil <cedric@deltheil.me>
108 lines
3.8 KiB
Python
108 lines
3.8 KiB
Python
from collections.abc import Sequence
|
|
from dataclasses import dataclass
|
|
from typing import Any, TypedDict
|
|
|
|
from jaxtyping import Bool
|
|
from torch import Tensor, nn
|
|
import numpy as np
|
|
import numpy.typing as npt
|
|
import torch
|
|
|
|
NDArrayUInt8 = npt.NDArray[np.uint8]
|
|
NDArray = npt.NDArray[Any]
|
|
|
|
|
|
class SAMInput(TypedDict):
|
|
image: Tensor
|
|
original_size: tuple[int, int]
|
|
point_coords: Tensor | None
|
|
point_labels: Tensor | None
|
|
boxes: Tensor | None
|
|
mask_inputs: Tensor | None
|
|
|
|
|
|
class SAMOutput(TypedDict):
|
|
masks: Tensor
|
|
iou_predictions: Tensor
|
|
low_res_logits: Tensor
|
|
|
|
|
|
class FacebookSAM(nn.Module):
|
|
image_encoder: nn.Module
|
|
prompt_encoder: nn.Module
|
|
mask_decoder: nn.Module
|
|
|
|
def __call__(self, batched_input: list[SAMInput], multimask_output: bool) -> list[SAMOutput]: ...
|
|
|
|
@property
|
|
def device(self) -> Any: ...
|
|
|
|
|
|
class FacebookSAMPredictor:
|
|
model: FacebookSAM
|
|
|
|
def set_image(self, image: NDArrayUInt8, image_format: str = "RGB") -> None: ...
|
|
|
|
def predict(
|
|
self,
|
|
point_coords: NDArray | None = None,
|
|
point_labels: NDArray | None = None,
|
|
box: NDArray | None = None,
|
|
mask_input: NDArray | None = None,
|
|
multimask_output: bool = True,
|
|
return_logits: bool = False,
|
|
) -> tuple[NDArray, NDArray, NDArray]: ...
|
|
|
|
|
|
@dataclass
|
|
class SAMPrompt:
|
|
foreground_points: Sequence[tuple[float, float]] | None = None
|
|
background_points: Sequence[tuple[float, float]] | None = None
|
|
box_points: Sequence[Sequence[tuple[float, float]]] | None = None
|
|
# TODO: support masks
|
|
# masks: Sequence[Image.Image] | None = None
|
|
|
|
def facebook_predict_kwargs(self) -> dict[str, NDArray]:
|
|
prompt: dict[str, NDArray] = {}
|
|
# Note: the order matters since `points_to_tensor` processes points that way (background -> foreground -> etc)
|
|
if self.background_points:
|
|
prompt["point_coords"] = np.array(self.background_points)
|
|
prompt["point_labels"] = np.array([0] * len(self.background_points))
|
|
if self.foreground_points:
|
|
coords = np.array(self.foreground_points)
|
|
prompt["point_coords"] = (
|
|
coords if "point_coords" not in prompt else np.concatenate((prompt["point_coords"], coords))
|
|
)
|
|
labels = np.array([1] * len(self.foreground_points))
|
|
prompt["point_labels"] = (
|
|
labels if "point_labels" not in prompt else np.concatenate((prompt["point_labels"], labels))
|
|
)
|
|
if self.box_points:
|
|
prompt["box"] = np.array([coord for batch in self.box_points for xy in batch for coord in xy]).reshape(
|
|
len(self.box_points), 4
|
|
)
|
|
return prompt
|
|
|
|
def facebook_prompt_encoder_kwargs(self, device: torch.device | None = None):
|
|
prompt = self.facebook_predict_kwargs()
|
|
coords: Tensor | None = None
|
|
labels: Tensor | None = None
|
|
boxes: Tensor | None = None
|
|
if "point_coords" in prompt:
|
|
coords = torch.as_tensor(prompt["point_coords"], dtype=torch.float, device=device).unsqueeze(0)
|
|
if "point_labels" in prompt:
|
|
labels = torch.as_tensor(prompt["point_labels"], dtype=torch.int, device=device).unsqueeze(0)
|
|
if "box" in prompt:
|
|
boxes = torch.as_tensor(prompt["box"], dtype=torch.float, device=device).unsqueeze(0)
|
|
points = (coords, labels) if coords is not None else None
|
|
# TODO: support masks
|
|
return {"points": points, "boxes": boxes, "masks": None}
|
|
|
|
|
|
def intersection_over_union(
|
|
input_mask: Bool[Tensor, "height width"], other_mask: Bool[Tensor, "height width"]
|
|
) -> float:
|
|
inter = (input_mask & other_mask).sum(dtype=torch.float32).item()
|
|
union = (input_mask | other_mask).sum(dtype=torch.float32).item()
|
|
return inter / union if union > 0 else 1.0
|