mirror of
https://github.com/finegrain-ai/refiners.git
synced 2024-09-20 03:15:26 +00:00
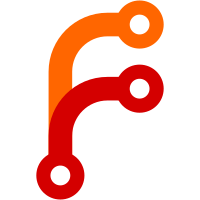
PyTorch chose to make it Any because they expect its users' code to be "highly dynamic": https://github.com/pytorch/pytorch/pull/104321 It is not the case for us, in Refiners having untyped code goes contrary to one of our core principles. Note that there is currently an open PR in PyTorch to return `Module | Tensor`, but in practice this is not always correct either: https://github.com/pytorch/pytorch/pull/115074 I also moved Residuals-related code from SD1 to latent_diffusion because SDXL should not depend on SD1.
114 lines
3.9 KiB
Python
114 lines
3.9 KiB
Python
import argparse
|
|
from pathlib import Path
|
|
|
|
import torch
|
|
from diffusers import UNet2DConditionModel # type: ignore
|
|
from torch import nn
|
|
|
|
from refiners.fluxion.model_converter import ModelConverter
|
|
from refiners.foundationals.latent_diffusion import SD1UNet, SDXLUNet
|
|
|
|
|
|
class Args(argparse.Namespace):
|
|
source_path: str
|
|
output_path: str | None
|
|
subfolder: str
|
|
half: bool
|
|
verbose: bool
|
|
skip_init_check: bool
|
|
|
|
|
|
def setup_converter(args: Args) -> ModelConverter:
|
|
# low_cpu_mem_usage=False stops some annoying console messages us to `pip install accelerate`
|
|
source: nn.Module = UNet2DConditionModel.from_pretrained( # type: ignore
|
|
pretrained_model_name_or_path=args.source_path,
|
|
subfolder=args.subfolder,
|
|
low_cpu_mem_usage=False,
|
|
)
|
|
source_in_channels: int = source.config.in_channels # type: ignore
|
|
source_clip_embedding_dim: int = source.config.cross_attention_dim # type: ignore
|
|
source_has_time_ids: bool = source.config.addition_embed_type == "text_time" # type: ignore
|
|
target = (
|
|
SDXLUNet(in_channels=source_in_channels) if source_has_time_ids else SD1UNet(in_channels=source_in_channels)
|
|
)
|
|
|
|
x = torch.randn(1, source_in_channels, 32, 32)
|
|
timestep = torch.tensor(data=[0])
|
|
clip_text_embeddings = torch.randn(1, 77, source_clip_embedding_dim)
|
|
|
|
target.set_timestep(timestep=timestep)
|
|
target.set_clip_text_embedding(clip_text_embedding=clip_text_embeddings)
|
|
added_cond_kwargs = {}
|
|
if isinstance(target, SDXLUNet):
|
|
added_cond_kwargs = {"text_embeds": torch.randn(1, 1280), "time_ids": torch.randn(1, 6)}
|
|
target.set_time_ids(time_ids=added_cond_kwargs["time_ids"])
|
|
target.set_pooled_text_embedding(pooled_text_embedding=added_cond_kwargs["text_embeds"])
|
|
|
|
target_args = (x,)
|
|
source_args = {
|
|
"positional": (x, timestep, clip_text_embeddings),
|
|
"keyword": {"added_cond_kwargs": added_cond_kwargs} if source_has_time_ids else {},
|
|
}
|
|
|
|
converter = ModelConverter(
|
|
source_model=source,
|
|
target_model=target,
|
|
skip_init_check=args.skip_init_check,
|
|
skip_output_check=True,
|
|
verbose=args.verbose,
|
|
)
|
|
if not converter.run(
|
|
source_args=source_args,
|
|
target_args=target_args,
|
|
):
|
|
raise RuntimeError("Model conversion failed")
|
|
return converter
|
|
|
|
|
|
def main() -> None:
|
|
parser = argparse.ArgumentParser(
|
|
description="Converts a Diffusion UNet model to a Refiners SD1UNet or SDXLUNet model"
|
|
)
|
|
parser.add_argument(
|
|
"--from",
|
|
type=str,
|
|
dest="source_path",
|
|
default="runwayml/stable-diffusion-v1-5",
|
|
help=(
|
|
"Can be a path to a .bin file, a .safetensors file or a model name from the HuggingFace Hub. Default:"
|
|
" runwayml/stable-diffusion-v1-5"
|
|
),
|
|
)
|
|
parser.add_argument(
|
|
"--to",
|
|
type=str,
|
|
dest="output_path",
|
|
default=None,
|
|
help=(
|
|
"Output path (.safetensors) for converted model. If not provided, the output path will be the same as the"
|
|
" source path."
|
|
),
|
|
)
|
|
parser.add_argument("--subfolder", type=str, default="unet", help="Subfolder. Default: unet.")
|
|
parser.add_argument(
|
|
"--skip-init-check",
|
|
action="store_true",
|
|
help="Skip check that source and target have the same layers count.",
|
|
)
|
|
parser.add_argument("--half", action="store_true", help="Convert to half precision.")
|
|
parser.add_argument(
|
|
"--verbose",
|
|
action="store_true",
|
|
default=False,
|
|
help="Prints additional information during conversion. Default: False",
|
|
)
|
|
args = parser.parse_args(namespace=Args())
|
|
if args.output_path is None:
|
|
args.output_path = f"{Path(args.source_path).stem}-unet.safetensors"
|
|
converter = setup_converter(args=args)
|
|
converter.save_to_safetensors(path=args.output_path, half=args.half)
|
|
|
|
|
|
if __name__ == "__main__":
|
|
main()
|