mirror of
https://github.com/Laurent2916/REVA-QCAV.git
synced 2024-09-19 19:45:28 +00:00
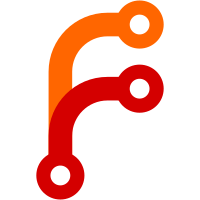
Former-commit-id: 84e2a715b843ecee2e12e4878fcee4a52bb0a4cb [formerly 1a5fc82bc099885853b7b4deff81b779dafd0168] Former-commit-id: c82cd66d6c432555a126e506631dfa2fd756437e
85 lines
2 KiB
Python
Executable file
85 lines
2 KiB
Python
Executable file
import argparse
|
|
import logging
|
|
|
|
import albumentations as A
|
|
import numpy as np
|
|
import torch
|
|
from albumentations.pytorch import ToTensorV2
|
|
from PIL import Image
|
|
|
|
from unet import UNet
|
|
|
|
|
|
def get_args():
|
|
parser = argparse.ArgumentParser(
|
|
description="Predict masks from input images",
|
|
)
|
|
parser.add_argument(
|
|
"--model",
|
|
"-m",
|
|
default="model.pth",
|
|
metavar="FILE",
|
|
help="Specify the file in which the model is stored",
|
|
)
|
|
parser.add_argument(
|
|
"--input",
|
|
"-i",
|
|
metavar="INPUT",
|
|
help="Filenames of input images",
|
|
required=True,
|
|
)
|
|
parser.add_argument(
|
|
"--output",
|
|
"-o",
|
|
metavar="OUTPUT",
|
|
help="Filenames of output images",
|
|
)
|
|
|
|
return parser.parse_args()
|
|
|
|
|
|
if __name__ == "__main__":
|
|
args = get_args()
|
|
|
|
logging.basicConfig(level=logging.INFO, format="%(levelname)s: %(message)s")
|
|
|
|
net = UNet(n_channels=3, n_classes=1)
|
|
|
|
device = torch.device("cuda" if torch.cuda.is_available() else "cpu")
|
|
logging.info(f"Using device {device}")
|
|
|
|
logging.info("Transfering model to device")
|
|
net.to(device=device)
|
|
|
|
logging.info(f"Loading model {args.model}")
|
|
net.load_state_dict(torch.load(args.model, map_location=device))
|
|
|
|
logging.info(f"Loading image {args.input}")
|
|
img = Image.open(args.input).convert("RGB")
|
|
|
|
logging.info(f"Preprocessing image {args.input}")
|
|
tf = A.Compose(
|
|
[
|
|
A.ToFloat(max_value=255),
|
|
ToTensorV2(),
|
|
],
|
|
)
|
|
aug = tf(image=np.asarray(img))
|
|
img = aug["image"]
|
|
|
|
logging.info(f"Predicting image {args.input}")
|
|
img = img.unsqueeze(0).to(device=device, dtype=torch.float32)
|
|
|
|
net.eval()
|
|
with torch.inference_mode():
|
|
mask = net(img)
|
|
mask = torch.sigmoid(mask)[0]
|
|
mask = mask.cpu()
|
|
mask = mask.squeeze()
|
|
mask = mask > 0.5
|
|
mask = np.asarray(mask)
|
|
|
|
logging.info(f"Saving prediction to {args.output}")
|
|
mask = Image.fromarray(mask)
|
|
mask.save(args.output)
|